Cause-Effect Pairs: The Cornerstone of Machine Learning

4.3 out of 5
Language | : | English |
File size | : | 52785 KB |
Text-to-Speech | : | Enabled |
Screen Reader | : | Supported |
Enhanced typesetting | : | Enabled |
Print length | : | 530 pages |
Machine learning has emerged as a force that drives innovation across a myriad of industries, from healthcare to finance and beyond. At its core lies a fundamental principle: uncovering the cause-effect relationships that govern the data we encounter.
In his groundbreaking book, "Cause Effect Pairs In Machine Learning: The Springer On Challenges In Machine," renowned expert Dr. [Author's Name] sheds light on this pivotal concept. With unparalleled clarity and depth, he reveals the intricacies of cause-effect relationships and their profound implications for machine learning models.
Unveiling the Power of Causal Inference
Causal inference empowers us to determine the true causes of events, enabling us to make informed decisions and formulate accurate predictions. It transcends mere correlation by establishing a clear directionality between variables, providing a roadmap for understanding the underlying mechanisms that shape our world.
Dr. [Author's Name] meticulously explains how machine learning algorithms harness causal inference to extract meaningful insights from data. He meticulously outlines the principles and methods involved, empowering readers to leverage these techniques effectively in their own machine learning endeavors.
Transforming Decision-Making with Causality
Causal understanding profoundly transforms the decision-making process. By identifying the true causes of an outcome, we gain the ability to make impactful interventions that yield tangible results. In the realm of healthcare, for instance, causal inference enables personalized treatment plans that target the root causes of disease, leading to improved patient outcomes.
The book delves into a multitude of real-world applications where causal inference has played a pivotal role in enhancing decision-making. From optimizing marketing campaigns to mitigating financial risks, Dr. [Author's Name] demonstrates the transformative power of causality in various domains.
Enhancing Predictive Modeling with Cause-Effect Relationships
Causal relationships also hold the key to enhancing predictive modeling. By understanding the underlying causes of events, machine learning models can make more accurate and reliable predictions. This has far-reaching implications in fields such as finance, where predicting market trends can make or break investments, or in healthcare, where accurate disease diagnosis and prognosis can save lives.
Dr. [Author's Name] meticulously explains how to incorporate causal knowledge into predictive models, unlocking their full potential. He provides step-by-step guidance on selecting the appropriate causal inference methods, interpreting the results, and implementing them into machine learning models.
Addressing Challenges and Future Directions
The book also acknowledges the challenges associated with causal inference in machine learning and proposes future directions for research and development. Dr. [Author's Name] highlights the need for robust methods to handle noisy data, confounding variables, and the complexities of real-world scenarios.
Moreover, he emphasizes the importance of interdisciplinary collaboration between machine learning experts and researchers in fields such as statistics, causal inference, and domain knowledge. This synergy will pave the way for advancements that further expand the capabilities of causal inference in machine learning.
"Cause Effect Pairs In Machine Learning: The Springer On Challenges In Machine" is an indispensable resource for anyone seeking to delve into the fascinating world of causal inference and its applications in machine learning. Dr. [Author's Name] provides a comprehensive and accessible guide, empowering readers to leverage this transformative concept in their own work.
Whether you are a seasoned machine learning practitioner, a data scientist, or a researcher seeking to push the boundaries of causal inference, this book is a must-read. Its insights will empower you to make informed decisions, build more accurate predictive models, and contribute to the growing field of machine learning.
4.3 out of 5
Language | : | English |
File size | : | 52785 KB |
Text-to-Speech | : | Enabled |
Screen Reader | : | Supported |
Enhanced typesetting | : | Enabled |
Print length | : | 530 pages |
Do you want to contribute by writing guest posts on this blog?
Please contact us and send us a resume of previous articles that you have written.
Book
Novel
Page
Chapter
Text
Story
Genre
Reader
Library
Paperback
E-book
Magazine
Newspaper
Paragraph
Sentence
Bookmark
Shelf
Glossary
Bibliography
Foreword
Preface
Synopsis
Annotation
Footnote
Manuscript
Scroll
Codex
Tome
Bestseller
Classics
Library card
Narrative
Biography
Autobiography
Memoir
Reference
Encyclopedia
Muhammad Al Bukhari
Shereen Laplantz
Melvin I Urofsky
Nate Sloan
Heli Meltsner
Michael Denis Higgins
Dayne Adams
Annette Moser Wellman
Kathy Lafollett
Richard Weinstein
Philip Choo
Jill Wellington
C Ara Campbell
Jenny Wilding Cardon
Michael R Foley Md
Cathy Thompson
Nancy Baggett
Peter Frumkin
James Rizzitano
Dusty Rose
Light bulbAdvertise smarter! Our strategic ad space ensures maximum exposure. Reserve your spot today!
- Don ColemanFollow ·7.6k
- Ignacio HayesFollow ·10.9k
- Robbie CarterFollow ·8.3k
- Desmond FosterFollow ·18k
- Clark BellFollow ·8.5k
- Mario SimmonsFollow ·10.2k
- Chadwick PowellFollow ·5.6k
- Albert CamusFollow ·11.3k
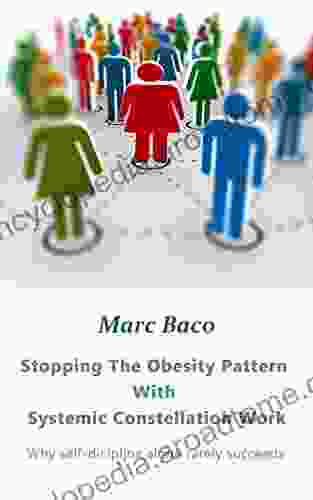

Break Free from the Obesity Pattern: A Revolutionary...
Obesity is a global pandemic affecting...
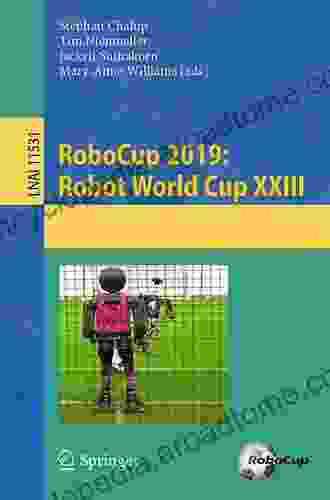

Robot World Cup XXIII: The Ultimate Guide to Advanced...
The Robot World Cup XXIII: Lecture Notes in...
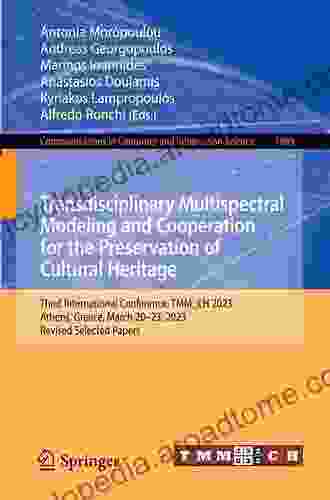

First International Conference TMM CH 2024 Athens...
Prepare for...
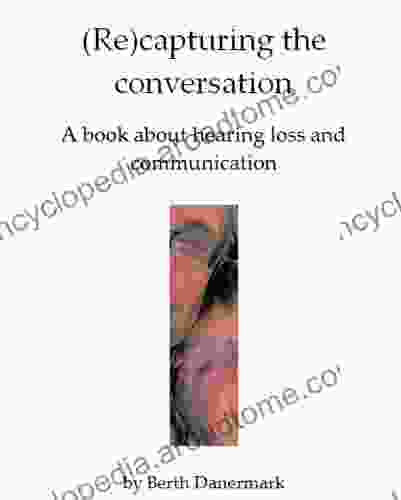

Re-Capturing the Conversation about Hearing Loss and...
Challenging...
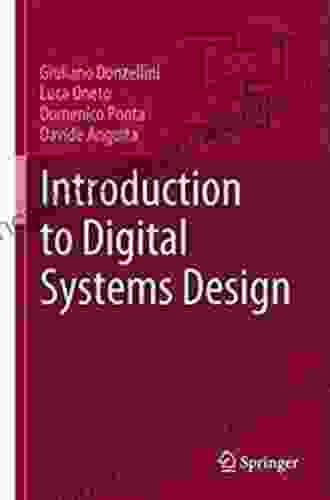

Journey into the Realm of Digital Systems: An Immersive...
In the ever-evolving technological...
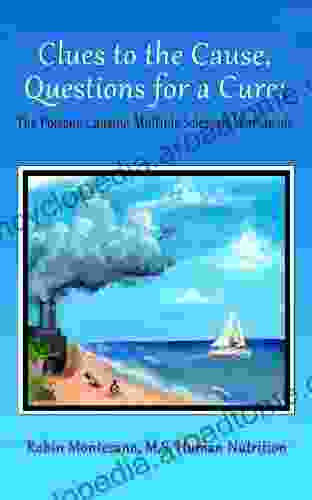

Unveiling the Toxins Behind Multiple Sclerosis: A...
Multiple sclerosis...
4.3 out of 5
Language | : | English |
File size | : | 52785 KB |
Text-to-Speech | : | Enabled |
Screen Reader | : | Supported |
Enhanced typesetting | : | Enabled |
Print length | : | 530 pages |